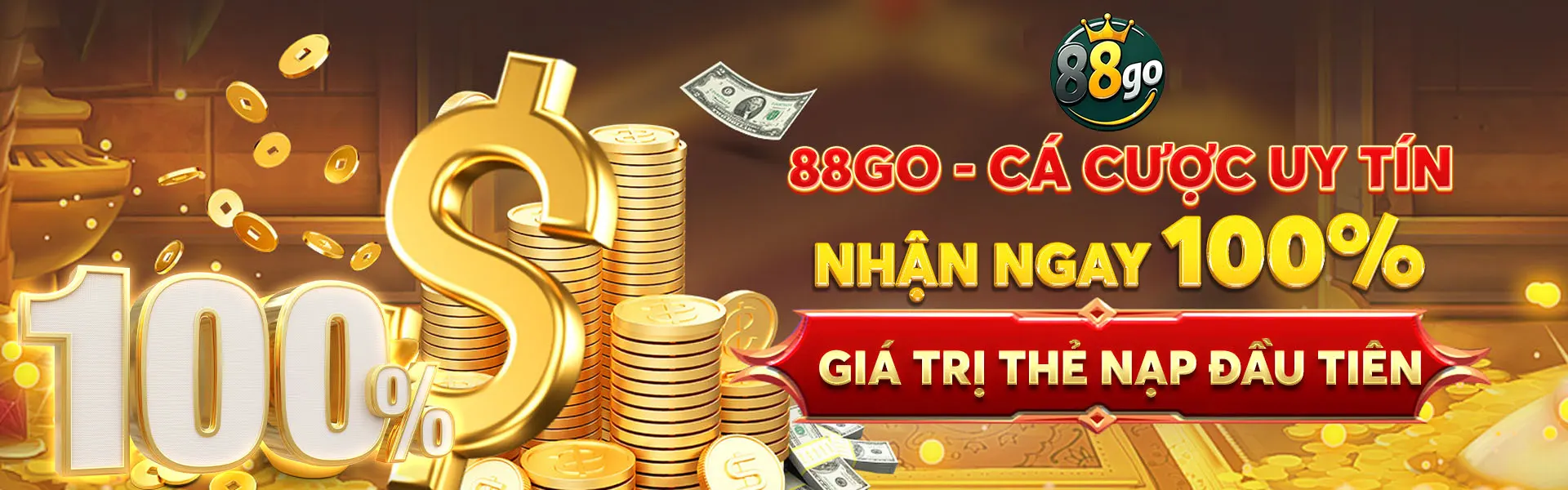
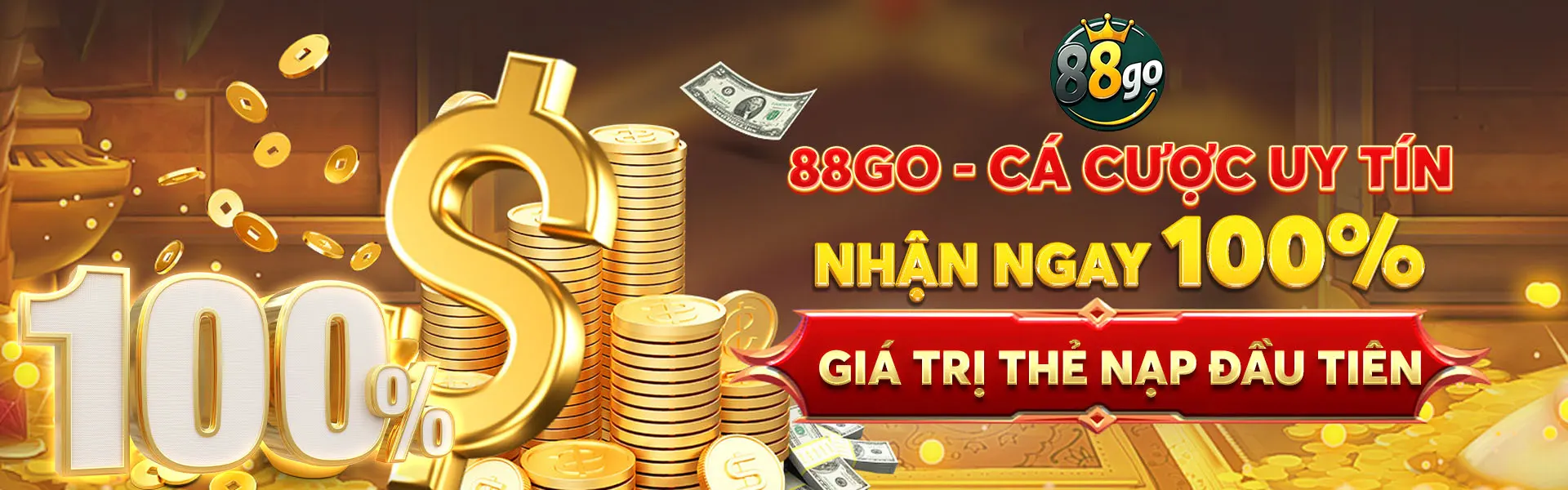
Chào mừng bạn đến với 88GO – trang cá cược hàng đầu tại Việt Nam! Chúng tôi tự hào mang đến những trò chơi hấp dẫn luôn được cập nhật thường xuyên, cùng nhiều chương trình khuyến mãi đặc biệt. Khi bạn nạp tiền lần đầu, bạn sẽ nhận ngay quà tặng hấp dẫn. Còn nhiều ưu đãi đáng chú ý đang chờ đón bạn trong những lần nạp tiếp theo! Hãy trải nghiệm cảm giác thú vị cùng 88GO ngay hôm nay! Để biết thêm thông tin chi tiết, hãy truy cập vào website chính thức của chúng tôi.
88GO – Link Vào Nhà Cái 88 GO Mới Nhất Hôm Nay 2025
ĐỐI TÁC NHÀ CÁI UY TÍN ĐẲNG CẤP